LDDMM FREE DOWNLOAD
This is crucial for clinical neuroimaging studies since the ultimate goal of any segmentation algorithm is to be able to accurately delineate brain structures with different diseases. Neuroimage 42 , — Methods of statistical shape analysis have proved useful for studying normal age related changes in subcortical nuclei and for studying a number of other diseases Bansal et al. Intersubject validation of linear and nonlinear models. The expectation-maximization EM algorithm yields the likelihood-fusion equation which is maximized by the a posteriori estimator of the segmentation labels.
Uploader: | Nehn |
Date Added: | 9 January 2013 |
File Size: | 19.86 Mb |
Operating Systems: | Windows NT/2000/XP/2003/2003/7/8/10 MacOS 10/X |
Downloads: | 16234 |
Price: | Free* [*Free Regsitration Required] |
Computational analysis of LDDMM for brain mapping
Six structures were analyzed left and right pairs of caudate, putamen and globus pallidus. Hippocampal morphometry in schizophrenia by high dimensional brain mapping. The other 4 were subjects with Alzheimer's disease AD mean DTIStudio Jiang et al. Psychiatry 64— Accuracy for each ROI dldmm quantified by three metrics. The fused likelihoods are modeled as conditional Gaussian random fields with mean fields a function of each atlas chart under its diffeomorphic change of coordinates onto the lddmm.
Support Center Support Center.
Large deformation diffeomorphic metric mapping
The multiple atlases with the associated convex weights imply that the posterior distribution is a multi-modal representation of the measured MRI. Imaging 30 oddmm, — Neuroimage 46— Regionally selective atrophy of subcortical structures in prodromal HD as revealed by statistial shape analysis.
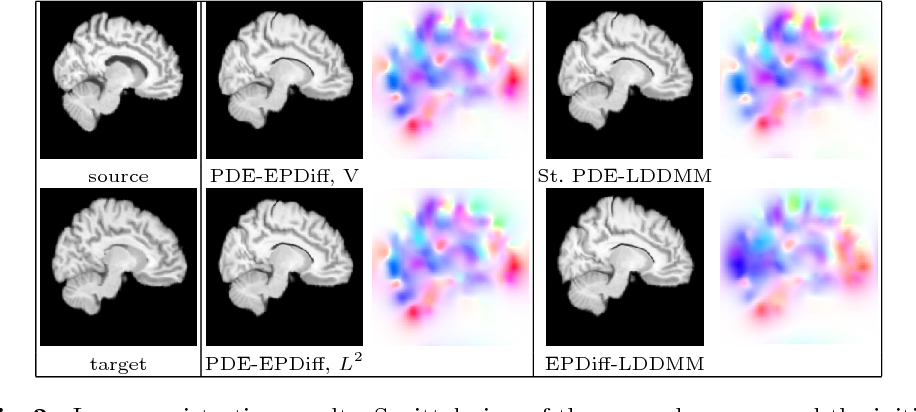
The theoretical framework we have adopted is based on the large deformation diffeomorphic metric mapping LDDMM algorithm Beg et al. Basal ganglia volume and shape in children with attention deficit hyperactivity disorder. To ensure that the solution lies in the space of diffeomorphisms, smoothness is achieved by defining the operator L as: Miller 1,7,8 and J. Accuracy is obtained at a cost of computational complexity.
Rather, in models based on Lagrangian and Eulerian flows of diffeomorphisms, the constraint is associated to topological properties, such as open sets being preserved, coordinates not crossing implying uniqueness and existence of the inverse mapping, and connected sets remaining connected.
Given an atlas image I 0 and a target image I 1which can be represented as functions I 0I 1: Geodesic Shooting for Computational Anatomy. Please discuss further on the talk page. LDDMM based segmentation is capable of achieving comparable or superior accuracy as measured by either the kappa statistic or the L 1 error.
Large Deformation Diffeomorphic Metric Mapping @ CIS
A new approach for unlabelled point-sets and sub-manifolds matching". This important distinction arises from the original formulation of the Riemannian metric corresponding to the right-invariance.
The variational problem matching onto: Miller1, 7, 8 and J. As in the original pipeline Miller and Qiu, in which segmentations were used to drive geodesic representations from which momentum and statistics were performed, the cascade approach described here allows us to generate intermediate analyses such as ldmdm in a computationally efficient way.
Methods of statistical shape analysis have proved useful for studying normal age related changes in subcortical nuclei and for studying a number of other diseases Bansal et al. Multiple comparisons were conducted using Tukey's HSD honestly significant difference test between pair wise groups. The charts and their diffeomorphic correspondences lddm unknown and viewed as latent or hidden variables. Further, proximity of sub-cortical structures can affect the accuracy and performance of automated segmentation algorithms resulting in conflicting results at the volumetric level Heckemann ,ddmm al.
Hsu2 Katarina A.
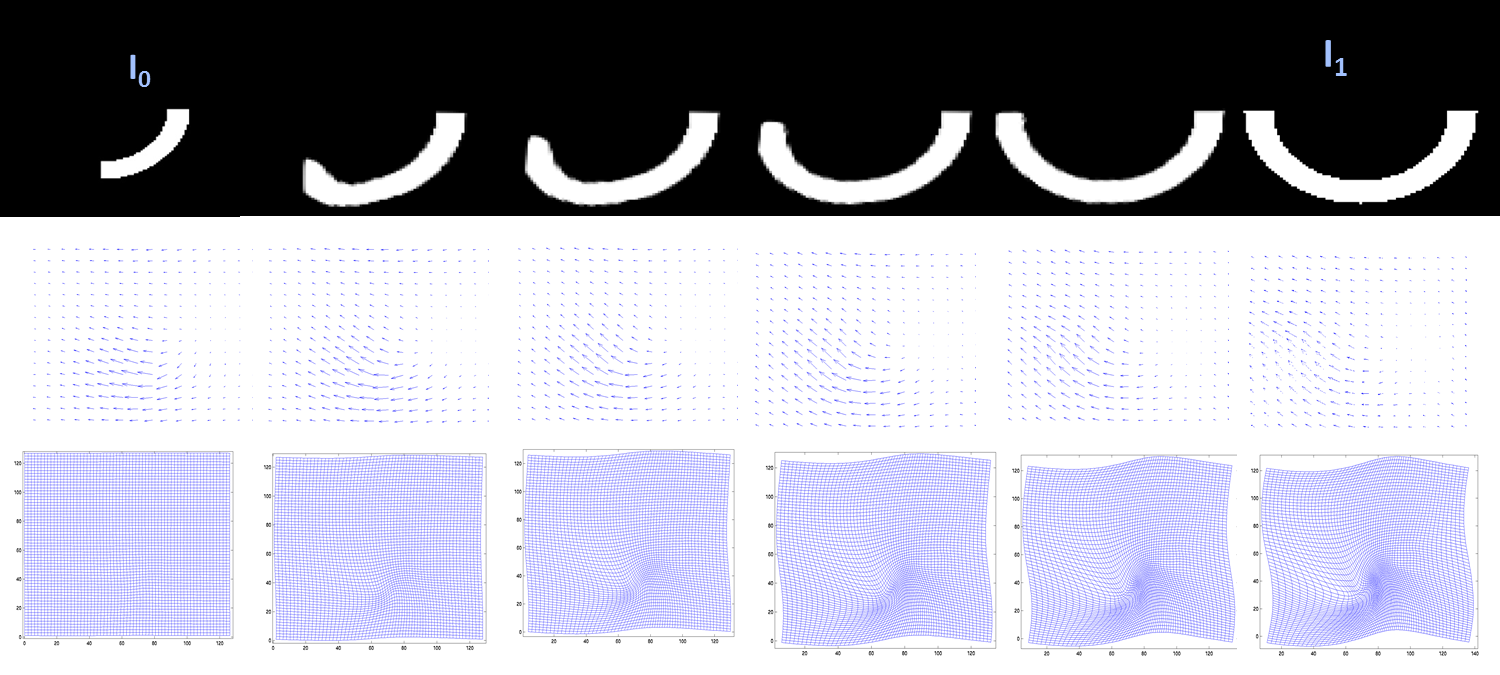
These large deformation methods have been extended to landmarks without registration via measure matching, [38] curves, [39] surfaces, [40] dense vector [41] and tensor [42] imagery, and varifolds removing orientation. Symmetric diffeomorphic image registration with cross-correlation: Beg solved the early LDDMM algorithms by solving the variational matching taking variations with respect to the vector fields.
This article has been cited by other articles in PMC. Diffeomorphic brain registration under exhaustive sulcal constraints. lrdmm
Comments
Post a Comment